Key Driver Analysis with Bayesian Networks — From Observational Data to Causal Inference
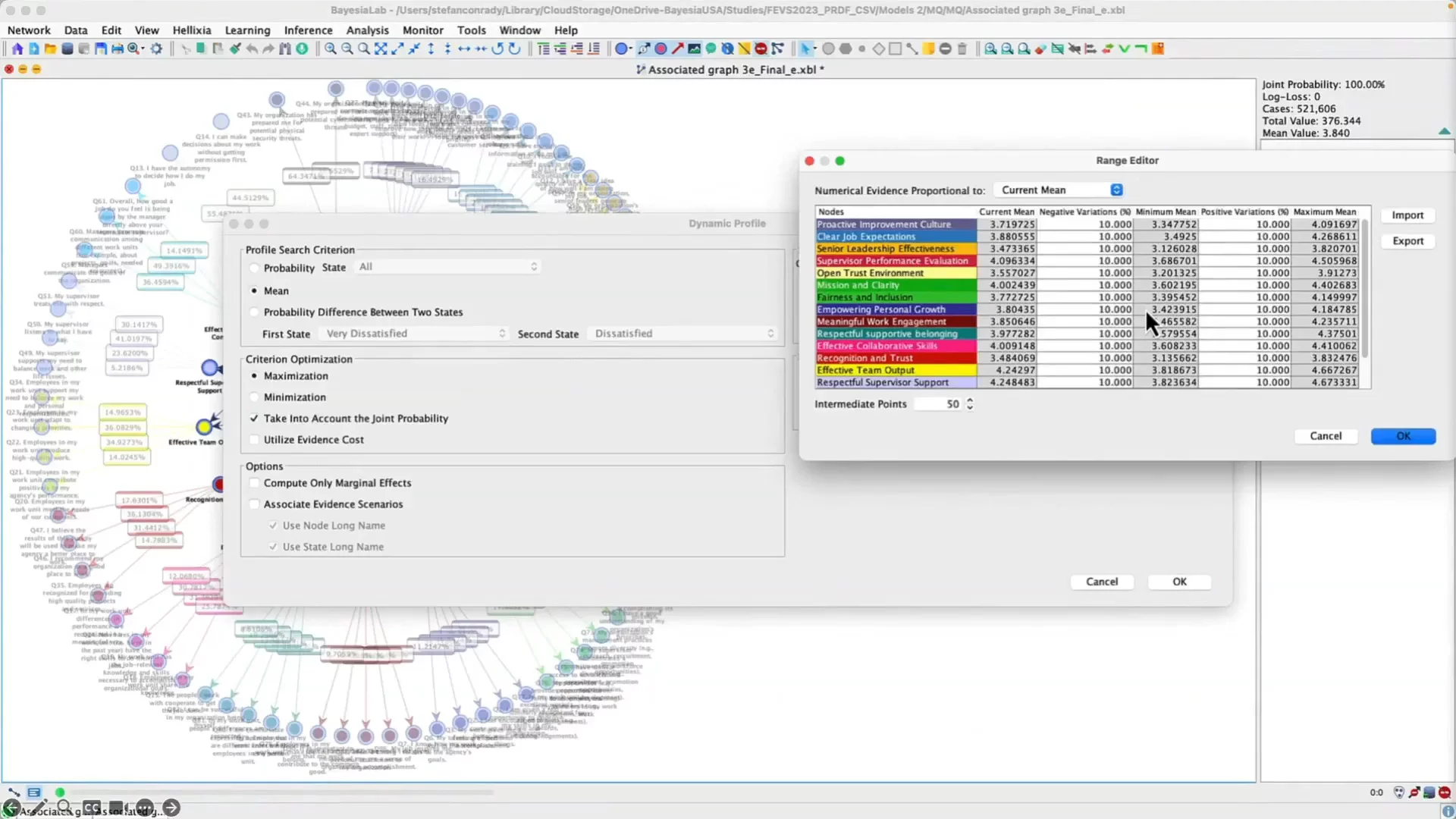
Bayesia
57:27
Please join us for a free live webinar on Thursday, April 24, 2025, at 11:00 a.m. (EDT, UTC-4), and learn about policy analysis and decision support with Bayesian networks and BayesiaLab.
Overview
Our free webinar offers a comprehensive tutorial on leveraging Bayesian networks, BayesiaLab, and GenAI for Key Driver Analysis and Decision Support. This webinar's case study focuses on HR Analytics, and we utilize data from the 2023 Federal Employee Viewpoint Survey (FEVS) to identify the optimal prioritization of management initiatives that would most effectively and efficiently enhance employee satisfaction. With years of consulting experience, we have successfully implemented this methodology for the world’s largest CPG, skincare, beverage, food, and healthcare companies.
Given the large number of variables in the survey, we begin our workflow by utilizing BayesiaLab's machine learning and clustering algorithms to create a Bayesian network model and reduce its dimensionality by identifying latent factors. Additionally, we use BayesiaLab's GenAI assistant, Hellixia, to generate meaningful characterizations of these newly identified factors.
Our first research objective is to prioritize opportunities for enhancing overall employee satisfaction at each federal agency by performing an observational Key Driver Analysis using the Bayesian network model learned from the FEVS data.
Next, we transition this machine-learned Bayesian network into a model designed for causal inference, enabling simulations of policy interventions, such as evaluating the effects of mandating a return to the office for employees. To support this shift from observational to causal inference, we incorporate domain knowledge into the model by applying the Disjunctive Cause Criterion.
This case study demonstrates how machine-learned Bayesian networks, combined with causal domain knowledge, can facilitate policy analysis for robust and audit-proof decision support.
Speaker
Stefan Conrady
Managing Partner, Bayesia USA
Stefan Conrady has over 20 years of experience in decision analysis, analytics, market research, and product strategy, having worked with Mercedes-Benz, BMW Group, Rolls-Royce Motor Cars, and Nissan across North America, Europe, and Asia.
As Managing Partner of Bayesia USA and Bayesia Singapore, he is widely recognized as a thought leader in applying Bayesian networks to research, analytics, and decision-making. Together with his business partner, Dr. Lionel Jouffe, he co-authored Bayesian Networks & BayesiaLab — A Practical Introduction for Researchers, an influential resource now widely cited in academic literature.
With their deep expertise in Bayesian networks for Key Driver Analysis and Optimization, Stefan and Lionel are highly sought-after consultants, advising global leaders such as Procter & Gamble, Coca-Cola, UnitedHealth Group, L’Oréal, the World Bank, and many of the world’s largest market research firms.
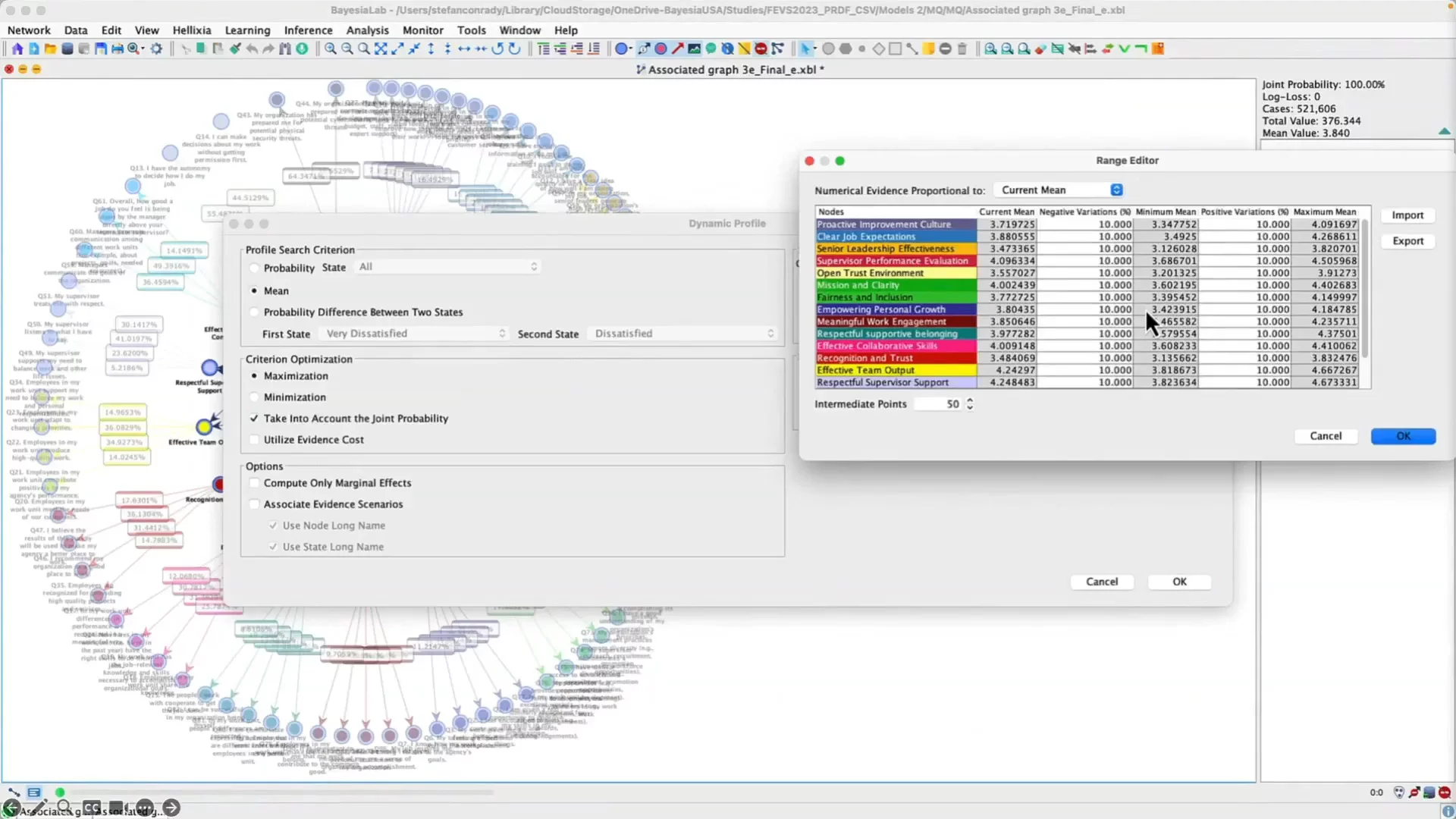
Key Driver Analysis with Bayesian Networks — From Observational Data to Causal Inference
57:27