Uncovering Hidden Patterns: The Power of Temporal Knowledge Graphs
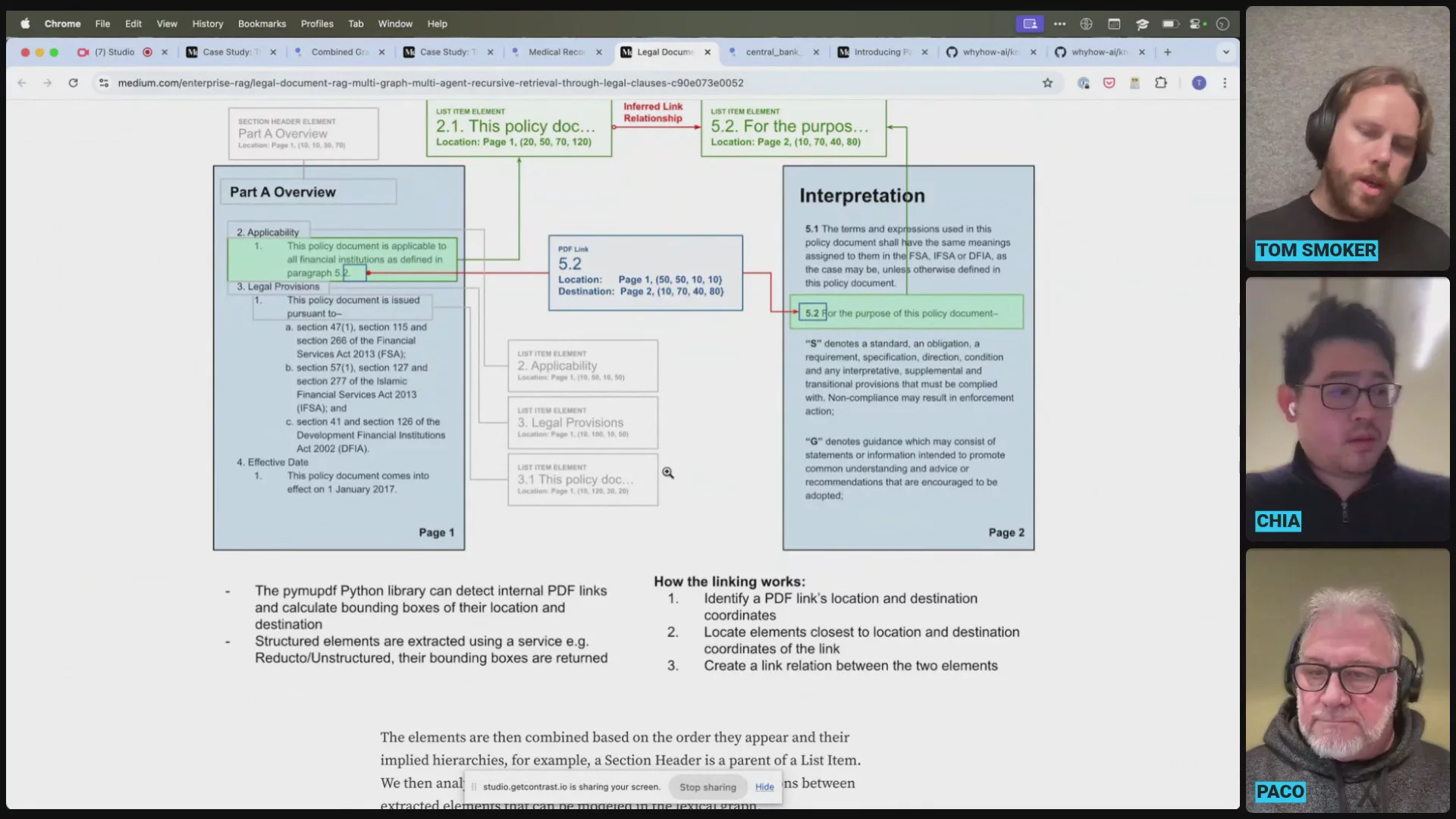
Senzing
01:07:41
Resources referenced in this session:
github.com/whyhow-ai/knowledge-table
github.com/whyhow-ai/knowledge-graph-studio
github.com/whyhow-ai/knowledge-graph-studiogithub.com/whyhow-ai/knowledge-table
synthetichealth.github.io/synthea/
hl7.org/fhir/patient.schema.json.html
Case Studies referenced in this session:
Extracting Insights From Unstructured Text
Temporal knowledge graphs provide new ways to extract insights from unstructured text data. By discovering relationships and dynamically identifying patterns, these graphs make it possible to track trends, identify key actors, and map shifts in topics or events.
Extracting entities, modeling how relationships change over time, and structuring the evolving data all require overcoming substantial technical hurdles. While temporal graphs offer significant theoretical promise, practical implementation reveals unexpected complexities—from managing incomplete time data to integrating models which keep pace with evolving information.
In this podcast, we’re joined by both Chia Jeng Yang and Tom Smoker from WhyHow.AI, as they share real-world lessons from constructing temporal knowledge graphs using congressional hearing transcripts. We’ll look at the practical techniques they used to transform messy, text-based data into meaningful, actionable knowledge, and discuss strategies for handling evolving datasets in research and analytics.
Why Attend?
- Gain practical insights into building and deploying temporal knowledge graphs.
- Learn strategies for extracting relationships and patterns from complex text data.
- Leverage state-of-the-art models for knowledge graph construction.
- Discover real-world applications for temporal graph modeling in research and analytics.
Speakers
Paco Nathan
Principal DevRel Engineer, Senzing
Paco Nathan leads DevRel for the Entity Resolved Knowledge Graph practice area at Senzing and is a computer scientist with +40 years of tech industry experience and core expertise in data science, natural language, graph technologies, and cloud computing. He's the author of numerous books, videos, and tutorials about these topics.
Tom Smoker
Technical Founder at WhyHow
Tom did his Masters in Software Engineering and PhD in Computer Science, specialising in NLP and knowledge graph embeddings in specialised domains. His post-academic career spans roles from data scientist to senior ML engineer at both large resource companies and startups. He applies his academic expertise to practical industry challenges, driving innovation in AI solutions across various sectors.
Chia Jeng Yang
Co-founder of WhyHow.AI
Chia is an ex-founder, operator and investor with a background from Cambridge/Harvard and spends his free time writing about LLMs at https://medium.com/enterprise-rag/.
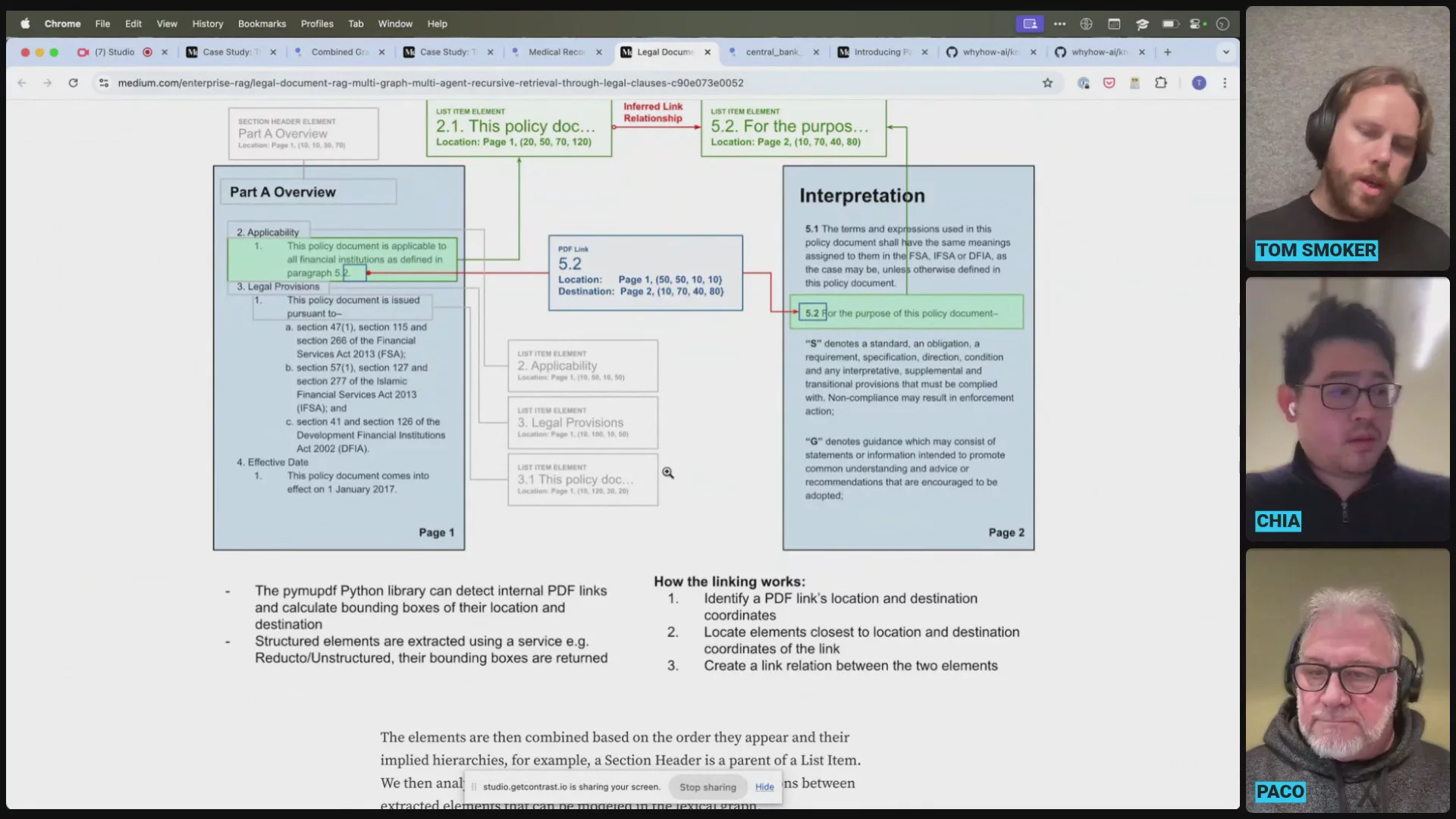
Uncovering Hidden Patterns: The Power of Temporal Knowledge Graphs
01:07:41