GraphRAG: The Good, the Bad, and the Ugly
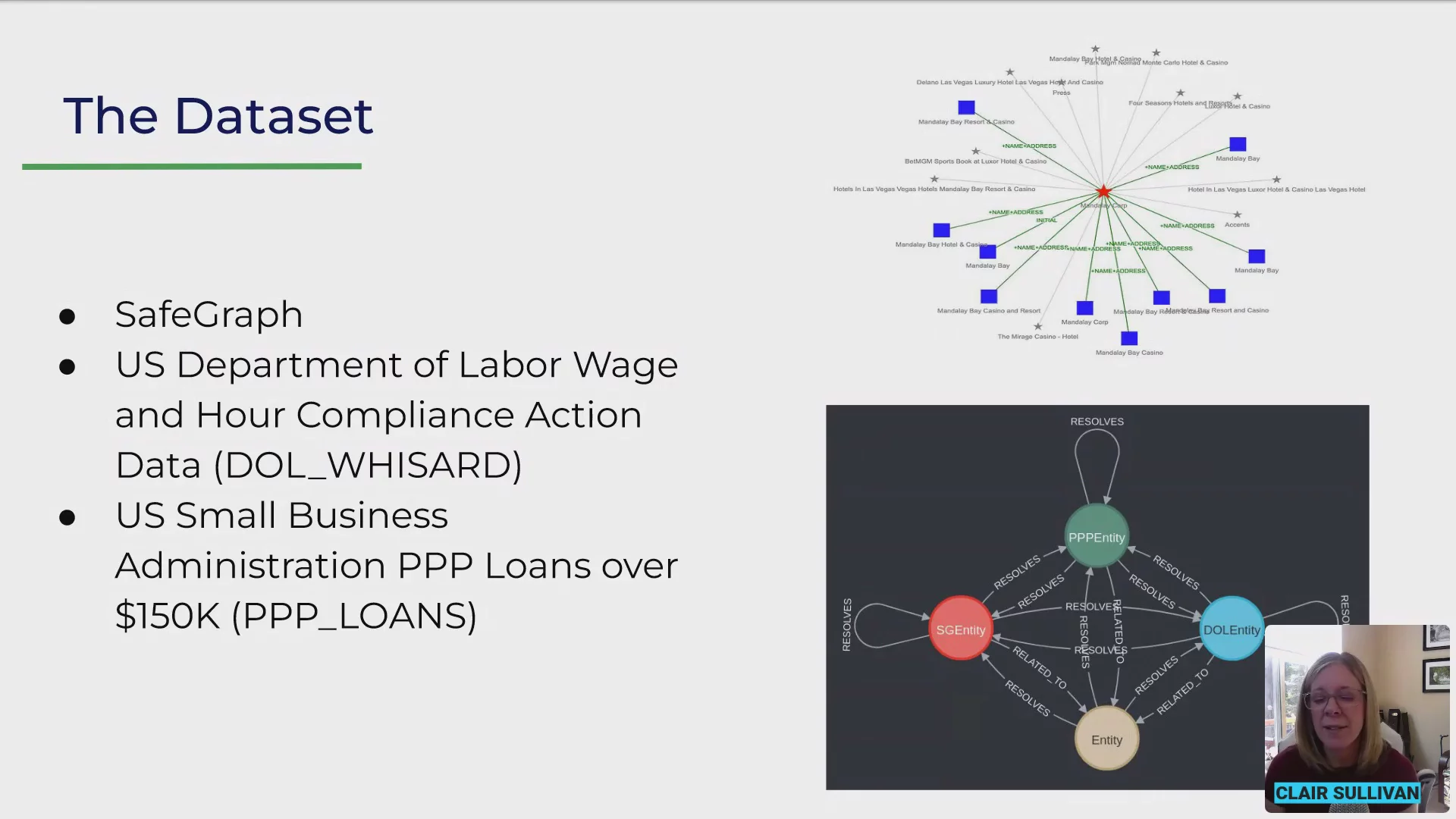
Senzing
01:13:30
Resources
Get the slides here
Visit Dr. Clair Sullivan's Github here
Read the Neo4 blog featuring: SafeGraph + WHISARD + PPP here
Read the guest blog for Senzing here
Dr. Clair Sullivan's tutorial here
Retrieval-Augmented Generation for AI-Generated Content: A Survey
Graphs are having a moment!
Thanks to the GenAI revolution, more people than ever are turning to graphs to solve problems like retrieval-augmented generation (RAG) and minimize hallucinations common in GenAI models. Graph structures excel at representing complex, interconnected data—critical for improving the contextual understanding that generative AI requires. By leveraging graphs, AI systems can better discover relationships, link entities, and enrich data, making them more effective at handling real-world complexity. As AI evolves, graphs are emerging as key enablers for more sophisticated, context-aware insights.
However, applying graphs to back GenAI applications is not without challenges. Extracting nodes and relationships from unstructured text via natural language processing (NLP) is difficult, as language is rich in context, idiomatic expressions, technical jargon, and implicit meanings that don’t neatly fit into graph structures. While large language models (LLMs) reflect general language usage, they often fail to capture subtle nuances and technical details, leading to oversimplified or inaccurate graph representations. This disconnect between the richness of language and the structured nature of graphs can hinder a system’s ability to generate significant insights.
In this talk, we will explore the use of graphs "from the trenches," examining real-world challenges encountered when applying them in complex systems. While graphs offer immense theoretical potential, translating that into practical applications often reveals unforeseen difficulties. From handling incomplete, ambiguous, or unresolved data to scaling large, interconnected datasets, the journey is rarely straightforward. We'll also discuss the challenges of integrating graphs with AI models, where the structured nature of graphs can clash with the messiness of real-world information. These practical insights will highlight the gap between the idealized use of graphs and the realities faced in the field.
Speakers
Paco Nathan
Principal DevRel Engineer, Senzing
Paco Nathan leads DevRel for the Entity Resolved Knowledge Graph practice area at Senzing and is a computer scientist with +40 years of tech industry experience and core expertise in data science, natural language, graph technologies, and cloud computing. He's the author of numerous books, videos, and tutorials about these topics.
Dr. Clair Sullivan
Founder and CEO
Dr. Clair Sullivan is currently the Founder and CEO of Clair Sullivan and Associates, a company dedicated to providing data science and generative AI freelance and consulting services. She received her doctorate degree in nuclear engineering from the University of Michigan in 2002. After that, she began her career in nuclear emergency response at Los Alamos National Laboratory where her research involved signal processing of spectroscopic data. She next spent 4 years working in the federal government on related subjects and returned to academic research in 2012 as an assistant professor in the Department of Nuclear, Plasma, and Radiological Engineering at the University of Illinois at Urbana-Champaign. While there, her research focused on using machine learning to analyze the data from large sensor networks. Deciding to focus more on commercial machine learning, she accepted a job at GitHub as a machine learning engineer while maintaining adjunct assistant professor status at the University of Illinois. In 2021 she joined Neo4j as a Graph Data Science Advocate. Prior to starting her current company, she was the Director of Data Science at Vail Resorts leading a team of data scientists and machine learning engineers providing production models for operations and marketing. She has authored 4 book chapters, over 20 peer-reviewed papers, and more than 30 conference papers. Dr. Sullivan was the recipient of the DARPA Young Faculty Award in 2014 and the American Nuclear Society's Mary J. Oestmann Professional Women's Achievement Award in 2015.
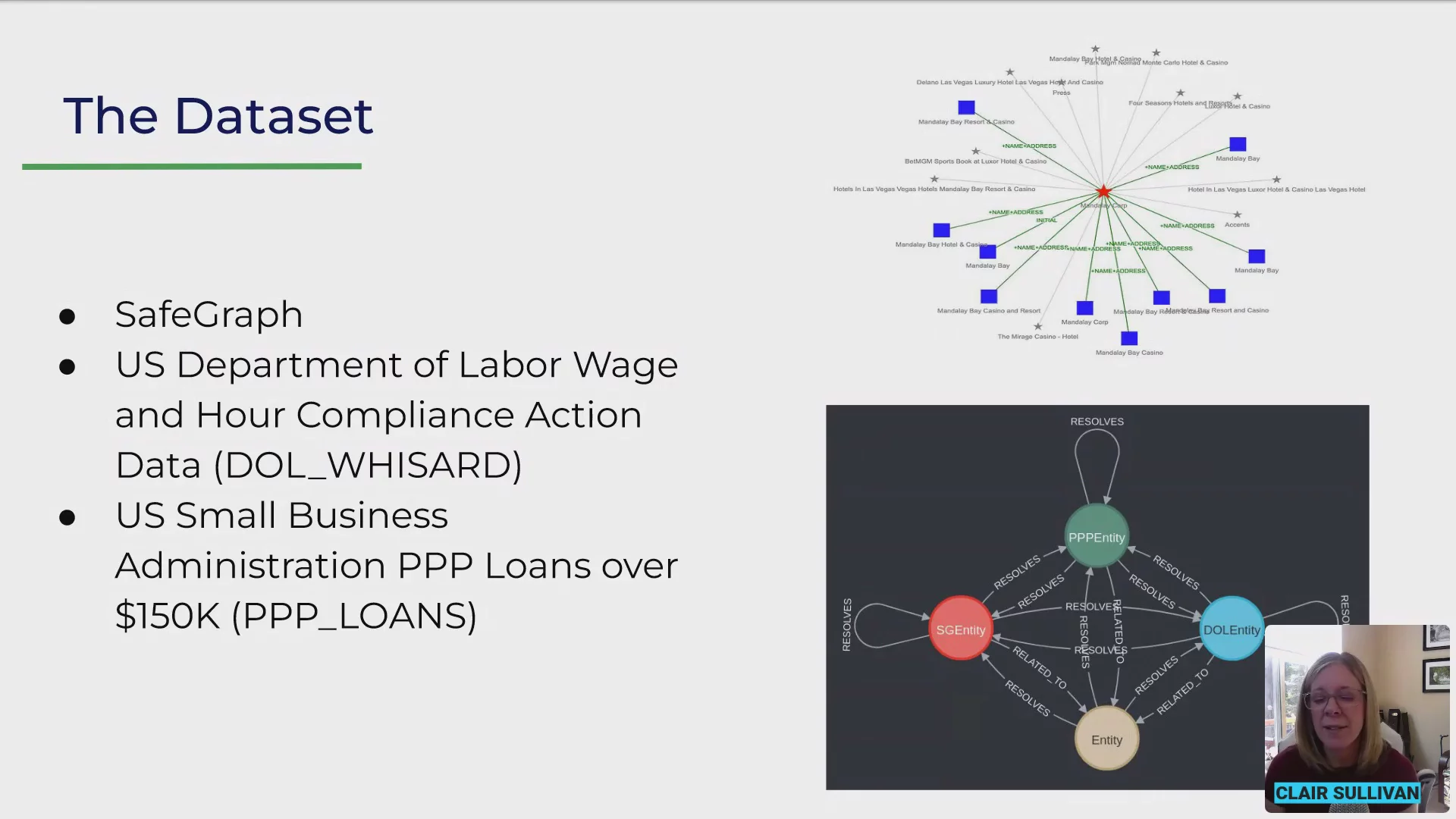
GraphRAG: The Good, the Bad, and the Ugly
01:13:30